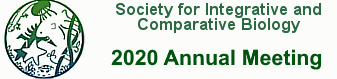
Meeting Abstract
An important component of fitness is the performance of a functional trait in its local environment. But environments vary spatially and temporally, and so too will the performance of the trait. The adaptive landscape of any given functional trait thus changes as an individual encounters different environments in its daily movements, as a local population experiences environmental change over time, as gene flow in a metapopulation crosses environmental gradients, or as descendant species colonize environments that are different from those occupied by their ancestors. Shifts in trait optima across these environmental boundaries creates a selectional trade-off that in some contexts favors a compromise trait value and in others changes the rate and direction of selection on the trait. Here I present a recently developed approach for modeling the effect of such trade-offs on the evolution of multivariate phenotypes. It uses geometric morphometrics to construct a continuous phenotypic space that defines the axes of the adaptive landscape. Differences in performance of phenotypes across that space are assessed using computational tools like finite element analysis (FEA) and computational fluid dynamics (CFD) or experimental strategies to estimate an adaptive landscape for each environmental situation. Using a combination of computer simulations and new data I show how maximum likelihood can be used to estimate the balance of environments that is most consistent with any given realized phenotype. I also show how this approach can be used in to predict the net phenotypic change expected from a shift in environment.