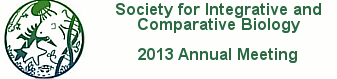
Meeting Abstract
67.1 Saturday, Jan. 5 Taking a step back: computational problems for morphological data revisited BRAZEAU, M.D.; Naturalis Biodiversity Center martin.brazeau@gmail.com
Although no longer as prevalent as molecular sequence data, morphological data remain an important source of phylogenetic and comparative data. Without them, it is impossible to place fossils in the tree of life or have a complete picture of the evolution of phenotypes. However, morphological characters are hierarchical, violating the assumptions of character independence in popular phylogenetic algorithms. Morphological character datasets may thus contain characters that are inapplicable to a subset of the taxa. Existing algorithms and software do not account for this, forcing researchers to develop workarounds at the level of character matrix construction. All these workarounds present computational problems. Possibly the most widely accepted approach to dealing with character inapplicability in morphological datasets is to score inapplicable taxa as missing data. However, it is well known that this approach may artificially add steps to tree scores, potentially discarding optimal solutions during the search process. Here I show modifications to the Fitch parsimony procedure that returns correct counts when non-applicable data are distinguished from missing data, but not considered a separate character state. A preliminary implementation examining published datasets shows that considerable resolution of published morphological trees may owe to computational artefacts owing to non-applicable data. The problem is further explored in light of parametric methods, such as maximum likelihood and Bayesian posterior probability, and possibilities for improving their applicability to morphological data. It is hoped that these considerations and developments can lead to improved algorithms for handling morphological data.