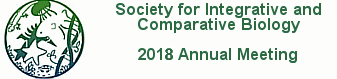
Meeting Abstract
Animals perform a variety of behaviors as they go about their daily lives. Biologists often think of these behaviors as being distinct from one another and the set of distinct behaviors an animal can execute is often referred to as that animal’s “behavioral repertoire.” Defining the behavioral repertoire of a given individual or species is a challenging and often subjective task. Here, we implement new unsupervised machine learning techniques to discover the behavioral repertoire of freely moving animals directly from tracking data. We further apply a dynamical machine learning approach to determine when animals transition from one distinct behavior to another using time series data. To demonstrate these methods, we apply them to data from a study of acoustically tagged salmon and salmon predators conducted in the Sacramento-San Joaquin River Delta, California. We first applied unsupervised machine learning for the quantitative classification of salmon and predator movement behavior from acoustic tagging data. This allows us not only to map the behavioral repertoires of the salmon and their predators, but also to define a transition probability of behavioral patterns between two observed time points. In the second step, we applied a statistical machine learning technique to identify predator attacks and predation events using a change-point detection algorithm. The change-point detection allows us to qualitatively determine if the behavior of the time series of the salmon changes and to identify the time point when this change occurs.