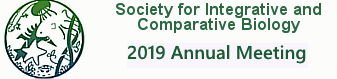
Meeting Abstract
Shifts in complex environments characterized by multiple co-varying factors present organisms unique challenges. The dynamic interplay among life-history traits, underlying physiological mechanisms, and the environment remains a fundamental, though poorly understood, issue. We quantified the effects of temperature (field-parameterized heat wave vs. control diel cycles), diet (low vs. high food availability), and immune activation (LPS challenge vs. no challenge) on parameters of life history (investment into growth, reproduction, and flight musculature) in the sand field cricket (Gryllus firmus), which exhibits a wing dimorphism that mediates a flight-fecundity tradeoff. We further quantified physiological parameters of total protein concentration and phenoloxidase activity, and metabolic rate. To predict how physiology and environments interactively influence life history, we used a hierarchy-aware unsupervised feature learning approach. We built a multilayer network where each layer represented interactions among individual life-history traits and physiology within different conditions (e.g., one layer was derived from individuals that experienced a heat wave, low food availability, and immune challenge): network nodes were linked across layers resulting in a multilayer network. An unsupervised machine learning algorithm was trained to automatically predict life-history trait values using features derived from the network. We also measured degree centrality, clustering coefficients, centrality scores, and diffusion to determine the relative importance of each parameter in the network to life history. We show that accounting for dynamic environmental variation and underlying physiology improves the power to predict variation in life-history traits linked to fitness.