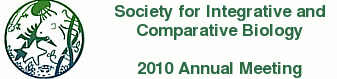
Meeting Abstract
64.2 Wednesday, Jan. 6 Machine Learning approaches to understanding the migratory behaviour of a small seabird, the Manx Shearwater (Puffinus puffinus) FREEMAN, R M*; DEAN, B; KIRK, H; PHILLIPS, R; ROBERTS, S; PERRINS, C; GUILFORD, T G; Animal Behaviour, Department of Zoology, Oxford Univeristy & Microsoft Research Cambridge; Animal Behaviour, Department of Zoology, Oxford Univeristy; Animal Behaviour, Department of Zoology, Oxford Univeristy; British Antarctic Survey, Cambridge; Information Engineering, University of Oxford; Edward Grey Institude, Department of Zoology, University of Oxford; Animal Behaviour, Department of Zoology, Oxford Univeristy robin.freeman@microsoft.com
Accurately determining the behaviour of individuals during their migration often requires expensive and relatively heavy telemetry devices. As such, research has necessarily focused on larger species and on smaller numbers of individuals. Here we explore the possibility of using data gathered from lower-cost, lightweight devices already widely deployed on smaller species (geolocators) to infer behaviour during migratory routes. Using methods from machine learning and pattern recognition to find patterns that correspond to those observed during summer foraging we are able to predict previously unseen marine stop-overs in a small Procellariiform, the Manx Shearwater (Puffinus puffinus). We will then discuss the potential for such analysis on wider datasets, explore the role of these interdisciplinary approaches and discuss some of our more recent findings.