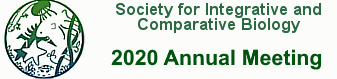
Meeting Abstract
Herbivorous leafcutter ants display an extreme polymorphism which is tied closely to task specialisation. However, studying such size-specific division of labour is a meticulous and error-prone task, as individual workers either have to be removed for weighing, or their weight has to be estimated from experience. To overcome these limitations, we developed a computer vision and machine learning-based tool which automatically detects and tracks variable numbers of ants within crowded areas such as foraging trails and sites, and simultaneously predicts their size and weight with high accuracy. This system consists of three independently trained deep convolutional neural networks. The first network robustly identifies individuals in crowded areas; it is a YoloV3 detector implemented in darknet, trained on a custom dataset to detect ants in various lighting conditions and degrees of occlusion. The second network is a newly developed modular buffer-and-recover tracking network which matches detections across frames, preventing identity switches with an accuracy of 99.2 %. The resulting tracks are then processed by a final deep convolutional regression network which estimates the weight of each individual. In contrast to previous implementations which were limited to a fixed number of individuals filmed in a well-defined environment, our marker-less detection system can cope with a time-variable number of individuals and does therefore not only significantly reduce the interference required for obtaining data, but also brings with it the potential to be used in the field. We demonstrate the potential of our tool by analysing differences in the worker size-frequency distribution of ants foraging on leaves of different mechanical properties, indicating tougher food sources being preferentially foraged on by larger workers.