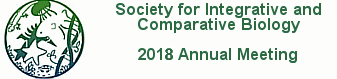
Meeting Abstract
Ecological niche modelling (ENM) is an emerging technique in modern macroecology for the quantitative prediction of species distributions based on abiotic environmental parameters. The potential to model the relationship between biological populations and multiple stressors in oceanographic systems makes this an attractive approach for predicting ecological responses to global change (at any timescale). The PaleoENM framework has laid the groundwork for the application of ecological niche modelling to the fossil record, opening an exciting research frontier for understanding the interactions between biodiversity and environmental changes in deep time. The paleontological record, unfortunately, does not directly record many of the abiotic environmental variables utilized by benthic marine ecology models in the modern ocean. In this study, we address the potential of the indirect environmental signals available in the geologic record to parametrize the ecological niche space of modern benthic macroinvertebrates by applying machine learning methods to large oceanographic and biodiversity datasets from the benthic ecosystems of Southern California. Random forest algorithms are employed to evaluate the potential of the geologic record to predict modern ecological distributions in the absence of direct oceanographic variables, focusing on bathymetry, sedimentology and geochemistry. Random forest and maximum entropy models are further applied to discrete ecological guilds to investigate the importance of different environmental variables to specific modes of animal life. Through this approach, we hope to advance understanding of how geologically available parameters relate to modern ecological processes and assess the proportion of ecologically relevant environmental information preserved in the geologic record.