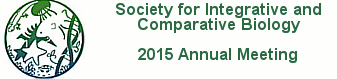
Meeting Abstract
Rapid, accurate, and inexpensive wood identification is an important factor in keeping illegal wood and wood products out of the supply chain, and thus combating illegal logging. U.S. enforcement of the Lacey Act and CITES treaty obligations have greatly increased the demand for wood identification expertise. Traditional human-mediated wood identifications, based upon pattern recognition of anatomical features, have proven too slow and too costly to meet the demands of law enforcement. To meet this demand, we have developed a portable machine vision system that can identify wood based upon an image of the transverse surface of an unknown specimen. Currently, the reference database includes 8000+ machine vision images of 160 species in 55 genera, a full order of magnitude greater taxonomic variation than any prior work. We capture a 2048- by 2048-pixel image of a 6.8- by 6.8-mm area of a transverse section of wood. Using this image, we compute discrete wavelet transform “energies” at 11 (log2[2048]) spatial scales within the image. Partial least squares discriminant analysis on these energies creates a classification system. The current system has a 60% success rate in identifying an unknown specimen, which is equal to or better than the average law enforcement officer with a week of training. Beyond wood identification, the plethora of images can be analyzed to explore anatomical characters at levels of quantitative detail hitherto not practical by traditional wood anatomy.