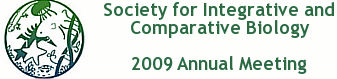
Meeting Abstract
63.1 Tuesday, Jan. 6 Bayesian Inference of Discrete Character States ORGAN, Chris*; MEADE, Andrew; PAGEL, Mark; Harvard University corgan@oeb.harvard.edu
Most evidence for understanding the biology of extinct organisms is absent from the fossil record. For example, evidence for behavior, genetics, and physiology rarely fossilize. Yet, as a primary research goal, paleontologists endeavor to reconstruct the biology of extinct organisms. Two parsimony methods have been available to paleontologists: the concentrated change test and phylogenetic bracketing. Neither account for uncertainty (in reconstructions or trees) and neither use the statistical correlation of one variable with a second variable to inform us about the state of the first in taxa with missing data (extinct species). The lack of branch length information in these approaches is another drawback, especially with bracketing because this method only provides qualitative tests of inference localized within a tree. We have previously reported a Bayesian method for inferring continuous characters given a tree and a dataset, which will be reviewed here. We report a new method for inferring binary character states. Our approach starts with an extant-only tree and estimates rates of character change, automatically testing for character correlation by reversible jump Markov-chain Monte Carlo that selects the most-likely model(s). We then include the extinct taxa and use the rate model(s) from the first step to infer states in the first character using MCMC given known states for the second character in the extinct taxa. Our approach uses information within the dataset, phylogenetic position, branch lengths, and estimated rates of character change to produce a posterior probability distribution of inferred states for the first character. It accounts for the uncertainty we have in both reconstructions and trees. Here we demonstrate the method using sex determination data for amniotes.