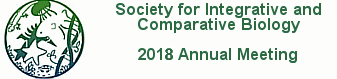
Meeting Abstract
A central biological principle is that cellular organization is strongly related to function. However, determining cellular organization is challenged by the multitude of different molecular complexes and organelles that comprise living cells and drive their behaviors. Currently, the experimental state-of-the-art for live cell imaging is limited to the simultaneous visualization of only a limited number (2-6) of tagged molecules. Modeling approaches can address this limitation by integrating subcellular structure data from diverse experiments. Generative models are useful in this context. They capture variation in a population and encode it as a probability distribution, accounting for the relationships among structures. However, these models suffer limitations, including dependence on preprocessing methods and limitations with respect to structures that vary widely in localization (diffuse proteins). Recent advances in adversarial networks (“deep learning”) are relevant to our problem. We present here a non-parametric (conditional generative) model of cell shape and nuclear shape and location, and relate it to the variation of other subcellular structures learned from live-cell, 3D microscopy images of our hIPSCs, gene edited with fluorescent reporters for the structure of interest. The model is trained on datasets of 100s-1000s of these fluorescence images and accounts for the spatial relationships among the intracellular structures of interest, their fluorescent intensities, and generalizes well to a variety of localization patterns. Using these relationships, the model allows us to predict the outcome of unobserved experiments, as well as encode complex image distributions into a low dimensional, probabilistic representation. This latent space serves as a compact coordinate system to explore variation.