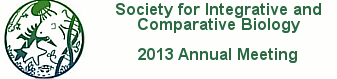
Meeting Abstract
25.1 Friday, Jan. 4 A biologically-based GIS model for predicting outbreaks of mosquito-borne viral diseases CARNEY, R.M.*; AHEARN, S.C.; MCCONCHIE, A; GLASER, C; JEAN, C; BARKER, C; PARK, B; PADGETT, K; PARKER, E; AQUINO, E; KRAMER, V; Brown University; City University of New York; University of British Columbia; California Dept of Public Health; California Dept of Public Health; Univ of California, Davis; Univ of California, Davis; California Dept of Public Health; California Dept of Public Health; California Dept of Public Health; California Dept of Public Health ryan_carney@brown.edu
The Dynamic Continuous-Area Space-Time (DYCAST) system is a biologically-based spatiotemporal model that uses public reports of dead birds to identify areas at high risk for West Nile virus (WNV) transmission to humans. We implemented this model prospectively using geographic information system (GIS) software during an unprecedented outbreak in California; daily risk maps were made available online and used by local agencies to target public education campaigns, surveillance, and mosquito control. DYCAST proved to be a timely and effective early warning system, with 80.8% sensitivity, 90.6% specificity, and identification of high-risk areas an average of 37.2 days prior to onset of illness. In subsequent years the model was implemented throughout the entire state of California. Additionally, we modified the model’s biological parameters based on the dengue infection cycle, and implemented an open-source version of the software retrospectively during a dengue epidemic in Riberão Preto, Brazil. Results indicate that the model provided early and accurate identification of high-risk areas, including the detection of cryptic interepidemic foci of transmission critical to efficacious control efforts. DYCAST predicted up to 90.3% (4,234/4,690) of cases, at a maximum mean of 66.3 days prior to onset of illness; sensitivity and specificity were 83.8% and 78.8%, respectively. Ultimately, this biological modeling approach has been shown to be an effective, inexpensive, and scalable solution for the surveillance and control of zoonotic diseases such as dengue and WNV, and has potential for further adaptation to model other ecological phenomena that cluster in space and time.