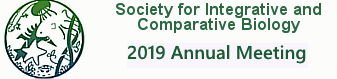
Meeting Abstract
Morphology -> performance relationships are well understood for individual performance traits, particularly in species that are highly specialized for conducting specific ecological tasks. However, the environment places multiple and often conflicting demands on organismal morphology and physiology. A proper understanding of the multivariate morphology -> performance relationship is currently lacking, at least in part because of logistical challenges in measuring multiple performance capacities on the same individuals. We developed and trained a set of machine learning models and compared their predictive performances based on cross-validation using existing morphology and performance for over 30 lizard species. We then combined some of the best performing models, in a stacking manner, and formed the final robust model to produce novel predictions of unmeasured performance traits in those same species. We consider the accuracy, validation, constraints, and shortcomings of the model, as well as several potential applications of this approach