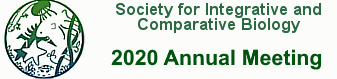
Meeting Abstract
Mathematical models are widely used to study animal behaviors. But for high-level tasks like sensorimotor processing, dynamics cannot be easily predicted by simple physical models. In these cases, data-driven system identification techniques are essential. This study focuses on system identification of refuge tracking responses in weakly electric glass knifefish, Eigenmannia virescens. During refuge tracking, fish swim forward and backward to track the movement of an actuated refuge. In these experiments, we command the refuge to follow one of two predefined input signals tuned to have similar overall signal power: (1) a sum of sinusoids comprising 13 single sine components with frequency of 0.1 Hz-2.05 Hz and (2) noise, filtered to 0 Hz-2.5 Hz. We use real time image processing software to record digitized refuge and fish trajectories. Given these input-output data, we use non-parametric system identification techniques to estimate the Frequency Response Function (FRF) of refuge tracking responses. To investigate how input signal selection affects FRF estimation, we performed an Empirical Transfer Function Estimate (ETFE) of the FRF. Preliminary results for N=2 fish suggest that ETFE performs reasonably well for the sum-of-sines stimulus, likely due to the high signal-to-noise ratio at a small number of frequencies, but produces erratic estimates for the pseudorandom input. Fortunately, FRF estimation for the noise stimulus is dramatically improved by introducing overlapped hamming windows to smooth time domain data. We find the improved noise input FRF estimation is similar to the result of deterministic input case, a critical validation that refuge tracking dynamics are approximately linear in this regime.