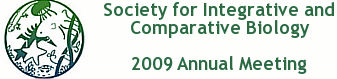
Meeting Abstract
P3.138 Tuesday, Jan. 6 Strategies for optimization in behavioral and ecological research using evolutionary computation ARTITA, Kimberly S.*; POLNASZEK, Timothy; SEARS, Michael W.; Southern Illinois University; Southern Illinois University; Southern Illinois University kartita@gmail.com
Classical models in behavioral ecology once were routinely solved using standard analytical techniques. As models have become more complex and have begun to include ever increasing levels of biological realism, such standard optimization techniques are often no longer sufficient methodologies. With the increased processing power of modern computers and with more easily-implemented distributed computing techniques, biologically-inspired computation has become feasible for solving models in behavioral ecology. Here, we implement and compare the performance of several biologically inspired techniques–simple genetic algorithm (SGA), particle swarm optimization (PSO), species conserving genetic algorithm (SCGA), and multispecies particle swarm optimization (MPSO)–to solve for optimal phenotypes with regard to probabilities of predator attack and prey flight in a predator-prey game. Although simple solutions to predict single phenotypes for predators and prey can be solved with SGA or PSO (with varying levels of efficiency), more interesting solutions that include additional alternative phenotypes potentially can be solved using SCGA or MPSO. In solving for optimal and alternative phenotypes in our predator-prey game, further simulations that compete each combination of predator and prey phenotype are required for model interpretation. The resulting solutions are analogous to those of classical game theory. Potential applications of this approach span a broad range of behavioral and ecological problems, especially those problems implemented at the individual level of organization.