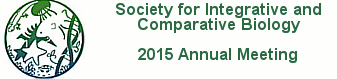
Meeting Abstract
A common application of ecological times series analysis is reconstruction of animal locations with classification of animal behaviors (e.g., foraging, migrating, aestivating) at those locations. Among the most common analytic tools for such data are dynamic state space models and change point analyses. We sought to analyze movements of sharks with a data set characterized by: 1) large numbers of observations (up to 16000 locations per individual, 2) frequent (> 100) putative behavior shifts, and 3) uneven time intervals between successive locations. These characteristics limited the utility of standard methods and we sought to extend state space models via a particle filtering/particle learning approach that we believe may be useful in other ecological applications. Our approach serially examines each successive measured location (assumed to be contaminated with measurement error), proposes a series of latent ‘true’ locations, ranks each path (particle) and preferentially selects those paths best corresponding to the measured locations as in the particle learning method of Carvahlo et al. The method is computationally intensive but allows both flexible description of the putative behavioral states (here foraging vs transiting) and confidence estimation for states at each location. Synthetic data sets suggest that state classifications can be improved by analyzing each data set repeatedly to eliminate estimates with low confidence, and finding ‘consensus’ estimates among high confidence estimates.