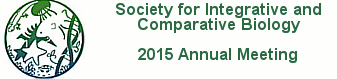
Meeting Abstract
Measuring behavioral activity budgets in small mammals is a difficult task due to its time-costliness, the difficulty of observing these animals in the wild, and the effects of observers on animal behaviors. In recent years tri-axial accelerometers have been increasingly employed to facilitate this task, however, their application in small mammals has been restricted, mainly due to weight limitations. Additionally, accelerometers engender a novel set of complications, due to the large datasets they produce and the need for a system that can reliably map acceleration patterns to specific behaviors. Using recently developed, tiny (1.5-2.0 g) accelerometers, we simultaneously collected filming and accelerometer data for two species of chipmunks (Tamias alpinus and Tamias speciosus) in a semi-naturalistic captive setting. We then manually labeled all films with behaviors in order to generate an accelerometer dataset annotated with specific behaviors. We then used techniques from machine learning to develop a system that can automatically label a novel stream of completely unprocessed accelerometer data with behavioral categories. Finally, we piloted this system in wild chipmunks, resulting in the collection and analysis of behavioral data from completely unobserved, free-living animals. This is a novel contribution to the field of remotely sensing behavioral activity budgets in small animals. Additionally, this study will open doors for an examination of behavioral differences in the focal species, which have divergently shifted their elevational ranges in response to the past century of climate change in Yosemite National Park, CA (Moritz et al., 2008).