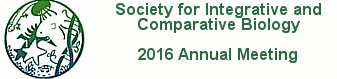
Meeting Abstract
Dimensionality reduction has been used to study locomotor and reaching biomechanics; however, such methods have had limited application to primate feeding, particularly to the hyolingual apparatus which features high functional variation. We applied both conventional and newer dimensionality reduction methods to a large feeding dataset to evaluate their ability to discriminate between two stages of feeding: chewing and swallowing. The dataset included the digitized anatomical and implanted markers of two adult rhesus macaques filmed in lateral view using videofluoroscopy while feeding on a variety of food items. The aggregated Cartesian coordinates of the markers constituted a quantified “posture” for each frame, and postures were analyzed using principal components analysis (PCA) and t-stochastic neighbor embedding (t-SNE). Preliminary results demonstrate that the first three principal components account for the majority of variation in posture (88%). Once embedded in low-dimensional space, pairwise distances among swallowing postures were smaller than a random sample from all cycles, and these distances were smaller in t-SNE than PCA. The results suggest that 1) intra-individual variation in feeding posture is low-dimensional and 2) dimensionality reduction techniques may be a useful tool for exploring large datasets of feeding biomechanics. Future directions will include using similar approaches to analyze datasets of three-dimensional motion capture data and electromyography of jaw, hyoid, and tongue musculature during feeding. Low-dimensional approaches may deepen our understanding of the major sources of variation in hyolingual behavior and how they contribute to feeding performance.