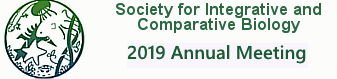
Meeting Abstract
Jellyfish are increasingly of interest to researchers due to their poorly understood behavior and ecosystem impacts. Understanding jellyfish locomotion and movement ecology has been hindered by the difficulty of attaching biologgers to gelatinous tissue. Previous biologging studies of jellyfish have used the “tether method” to recover tags, in which tagged animals are attached to drifters transmitting location information. Although this method imposes restrictions on the animal’s movement, its precise effects have never been quantified, thereby compromising research findings. Here we develop novel machine learning methods to 1) identify uninfluenced portions of the tag data and 2) classify fine-scale jellyfish behavior. We deployed the ITAG, a biologging package with motion and environmental sensors, on 8 Chrysaora fuscescens in Monterey Bay. By collecting simultaneous video footage of the tagged organisms with a remotely operated vehicle, we were able to train machine learning classifiers to identify periods of tether influence and animal swimming, drifting, and turning. We used these tools to (1) characterize the heading over time of C. fuscescens relative to a drogued drifter, (2) estimate in situ jellyfish activity, finding that animals actively swim more than 97.5% of the time, and (3) deduce that turning is more energetically costly than straight swimming. Our work demonstrates how machine learning methods can help maximize the insights yielded by biologging, and contributes new techniques for understanding fine-scale in situ animal behavior.